(# for the corresponding author, * for equal contribution)
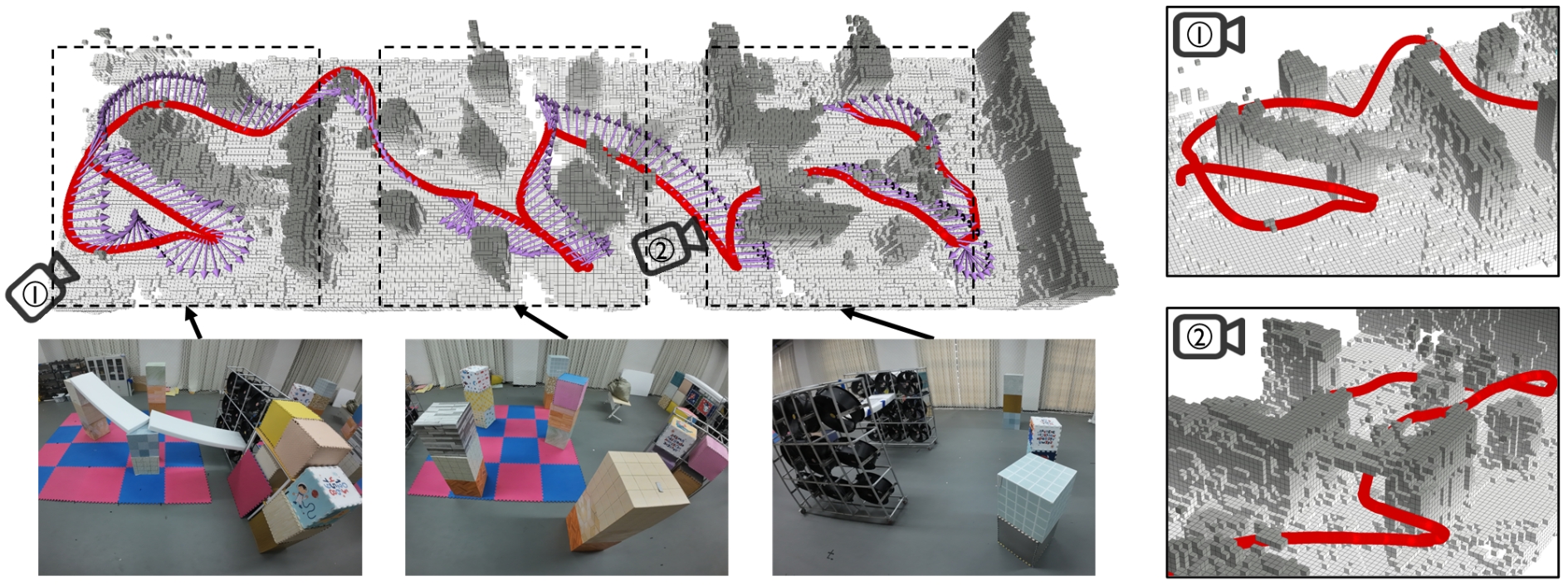 |
FALCON: Fast Autonomous Aerial Exploration using Coverage Path Guidance
Yichen Zhang*, Xinyi Chen*, Chen Feng, Boyu Zhou#, and Shaojie Shen.
[Under Review] Submitted to IEEE Transactions on Robotics (T-RO), 2024.
This paper introduces FALCON, a novel Fast Autonomous expLoration framework using COverage path guidaNce, which aims at setting a new performance benchmark in the field of autonomous aerial exploration. FALCON effectively harnesses the full potential of online generated coverage paths in enhancing exploration efficiency.
|
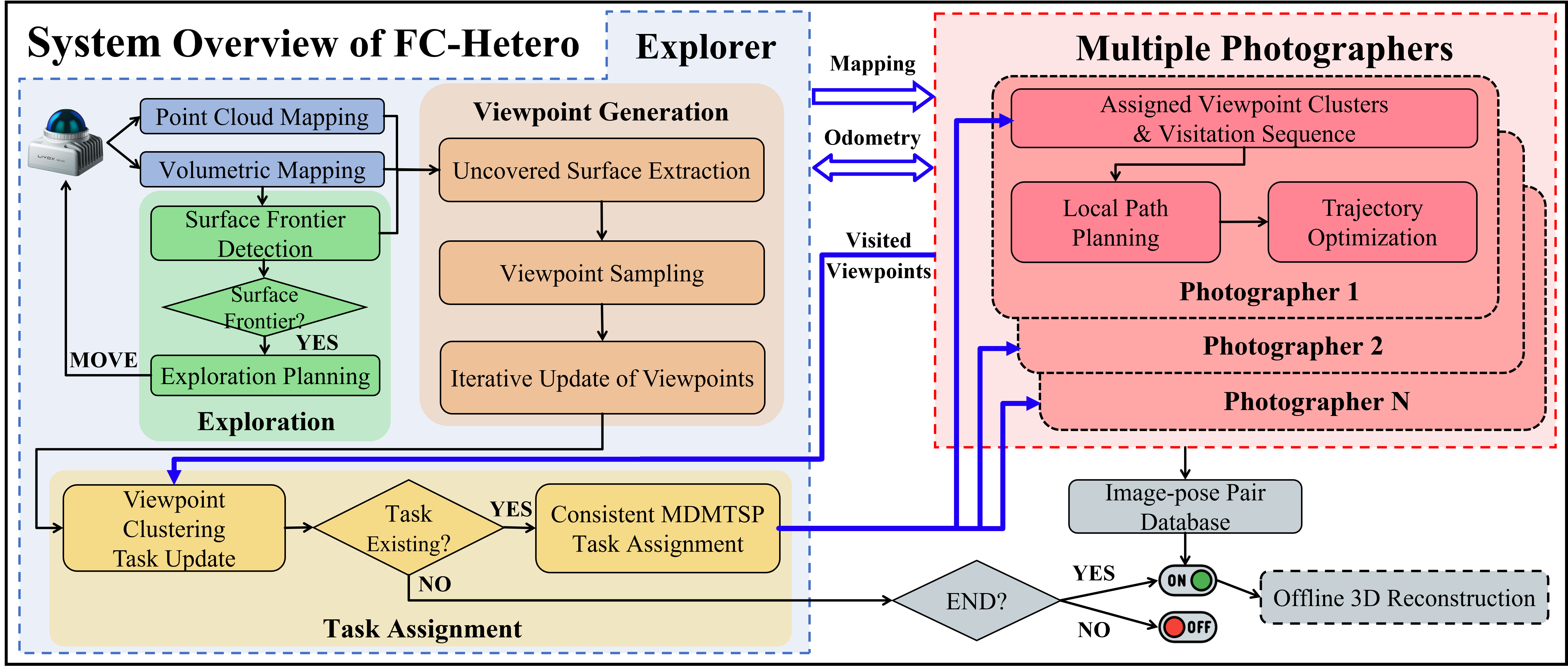 |
FC-Hetero: Fast and Autonomous Aerial Reconstruction Using a LiDAR-Visual Heterogeneous Multi-UAV System
Mingjie Zhang*, Chen Feng*, Zengzhi Li, Guiyong Zheng, Yiming Luo, Zhu Wang, Jinni Zhou, Shaojie Shen, and Boyu Zhou#.
IEEE/RSJ International Conference on Intelligent Robots and Systems (IROS),
2024. (Oral, acceptance rate: 10%)
Star
We introduce FC-Hetero, a LiDAR-visual heterogeneous UAV Multi-UAV system that can support fast aerial coverage and reconstruction in complex environments.
|
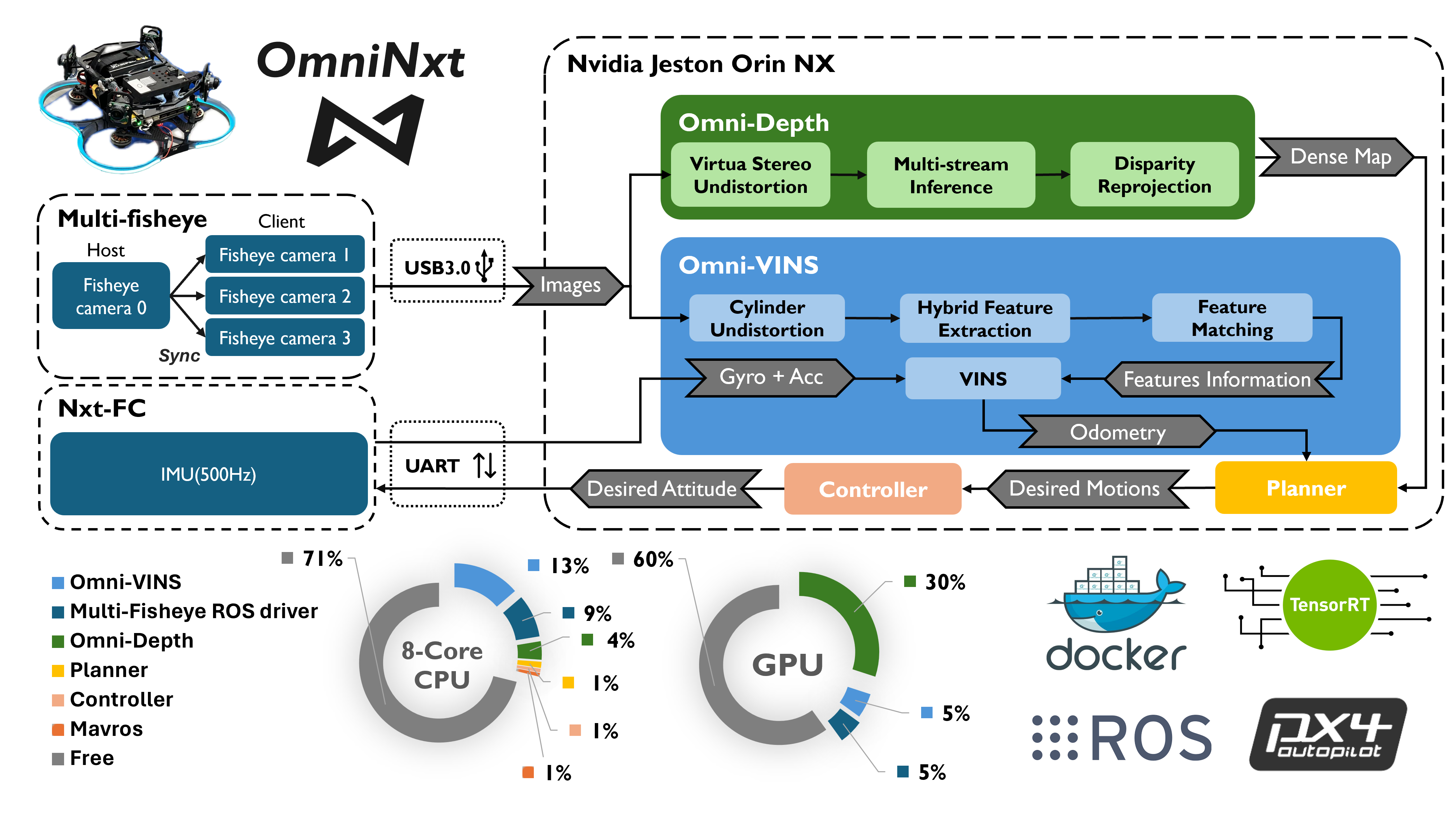 |
OmniNxt: A Fully Open-source and Compact Aerial Robot with Omnidirectional Visual Perception
Peize Liu, Chen Feng#, Yang Xu, Yan Ning, Hao Xu#, and Shaojie Shen.
IEEE/RSJ International Conference on Intelligent Robots and Systems (IROS),
2024. (Oral, acceptance rate: 10%)
Star
We introduce OmniNxt, the first fully open-source aerial robotics platform with omnidirectional visual perception. OmniNxt boasts a compact size with exceptional computational resources. Thanks to our meticulous development, we endow OmniNxt with an expansive perception range and efficient resource consumption.
|
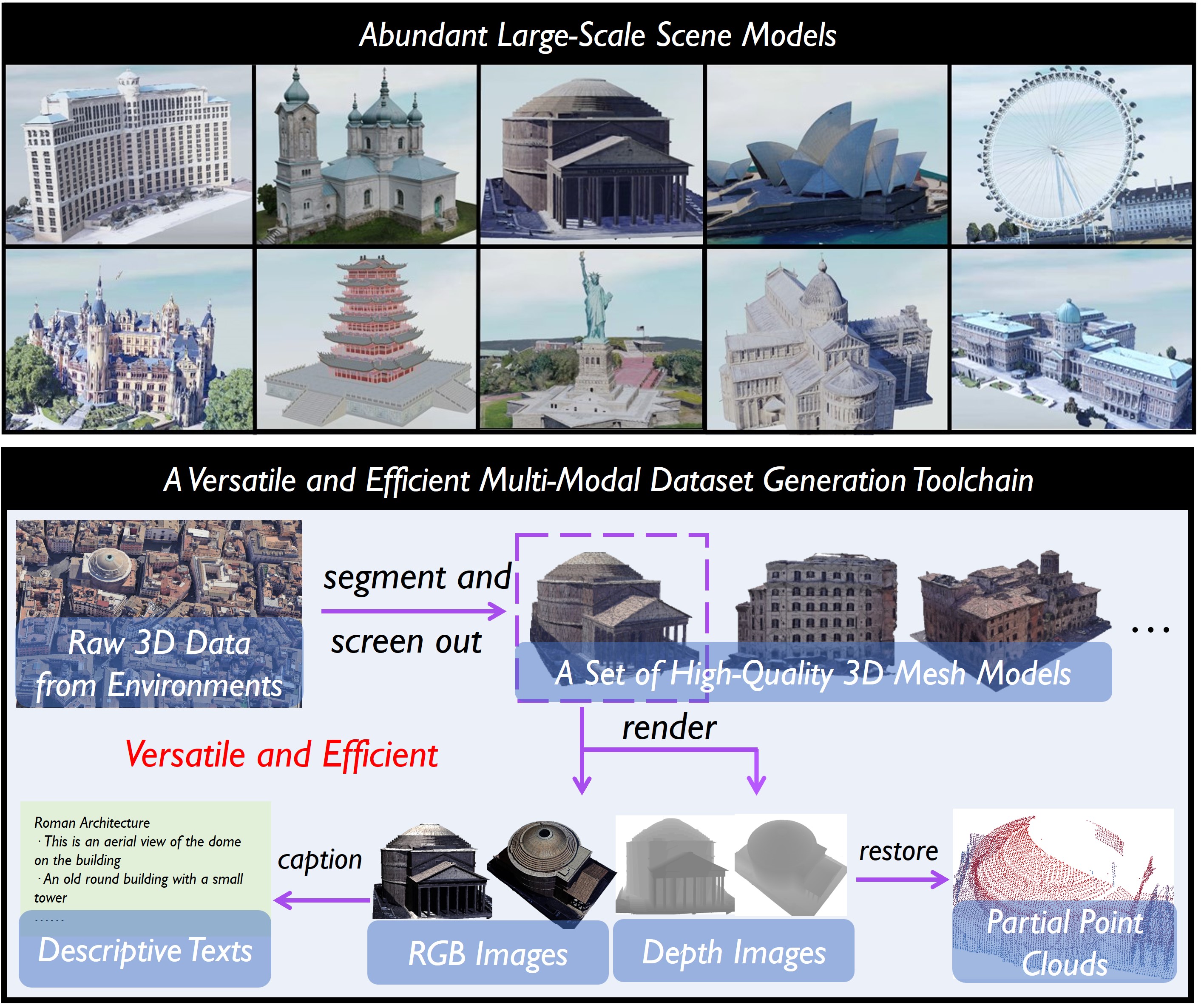 |
MASSTAR: A Multi-Modal Large-Scale Scene Dataset with a Versatile Toolchain for Surface Prediction and Completion
Guiyong Zheng*, Jinqi Jiang*, Chen Feng*, Shaojie Shen, and Boyu Zhou#.
Tech Report, 2024.
Star
We propose MASSTAR, a Multi-modal lArge-scale Scene dataset with a verSatile Toolchain for surfAce pRediction and completion. We develop a versatile and efficient toolchain for processing the raw 3D data from the environments.
|
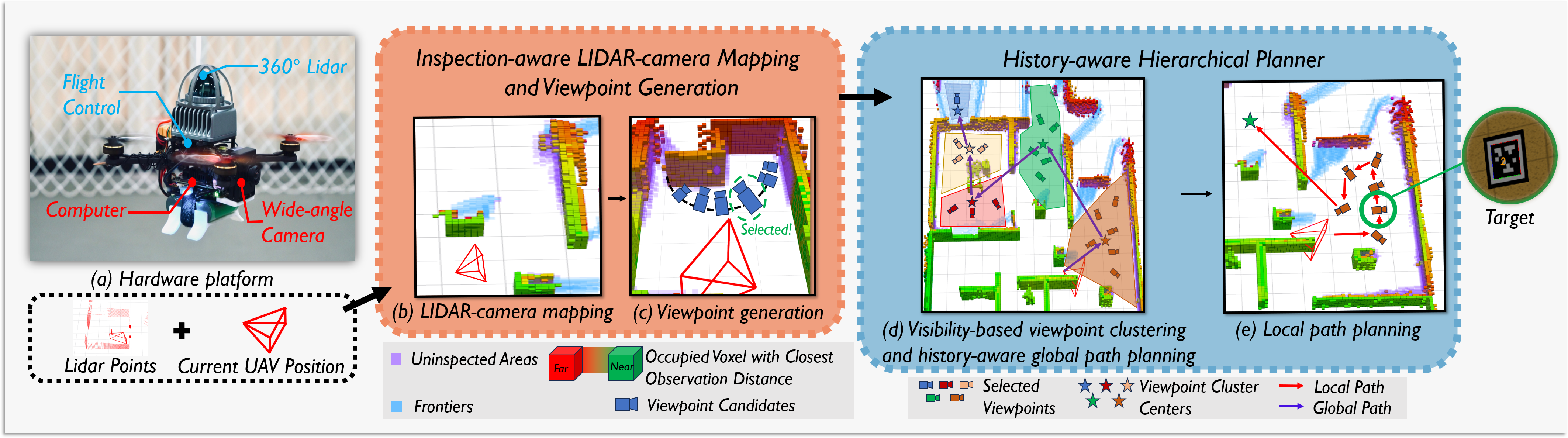 |
Star-Searcher: A Complete and Efficient Aerial System for Autonomous Target Search in Complex Unknown Environments
Yiming Luo, Zixuan Zhuang, Neng Pan, Chen Feng, Shaojie Shen, Fei Gao, Hui Cheng, and Boyu Zhou#.
IEEE Robotics and Automation Letters (RA-L) with IROS, 2024.
Star
We propose Star-Searcher, a complete and efficient aerial system for
autonomous target search in complex unknown environments. Our aerial system incorporates specialized sensor suites, mapping, and planning modules, all geared towards
improving task efficiency and completeness.
|
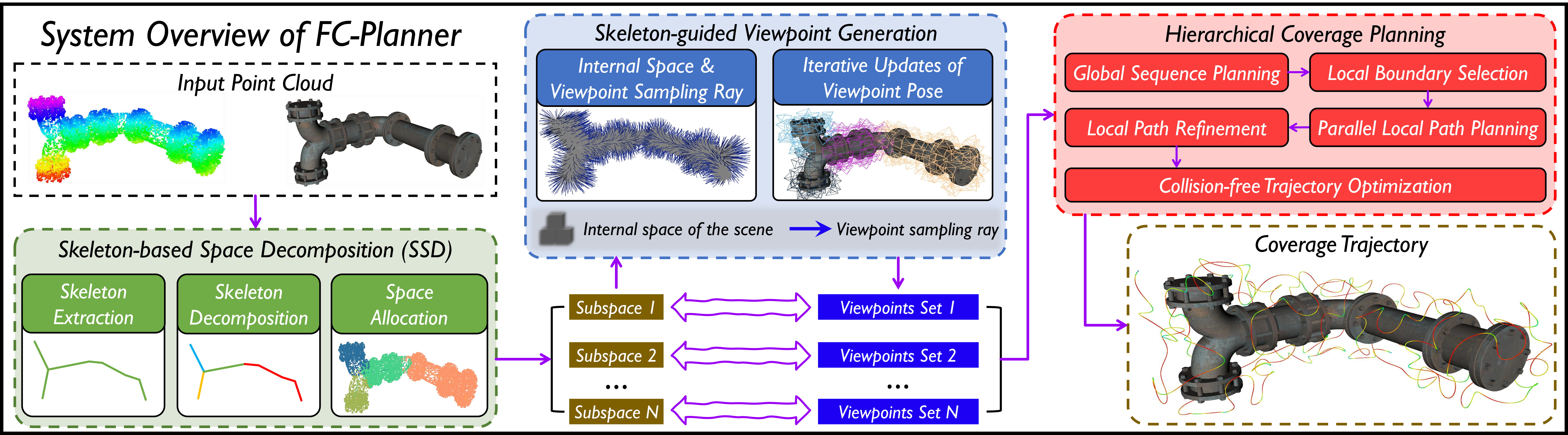 |
FC-Planner: A Skeleton-guided Planning Framework for Fast Aerial Coverage of Complex 3D Scenes
Chen Feng, Haojia Li, Mingjie Zhang, Xinyi Chen, Boyu Zhou#, and Shaojie Shen.
IEEE International Conference on Robotics and Automation (ICRA),
2024. (Best UAV Paper Award Finalist)
Star
We propose FC-Planner, a skeleton-guided planning framework tailored for fast coverage
of large and complex 3D scenes, which results in the generation of high-quality coverage paths and high computational efficiency.
|
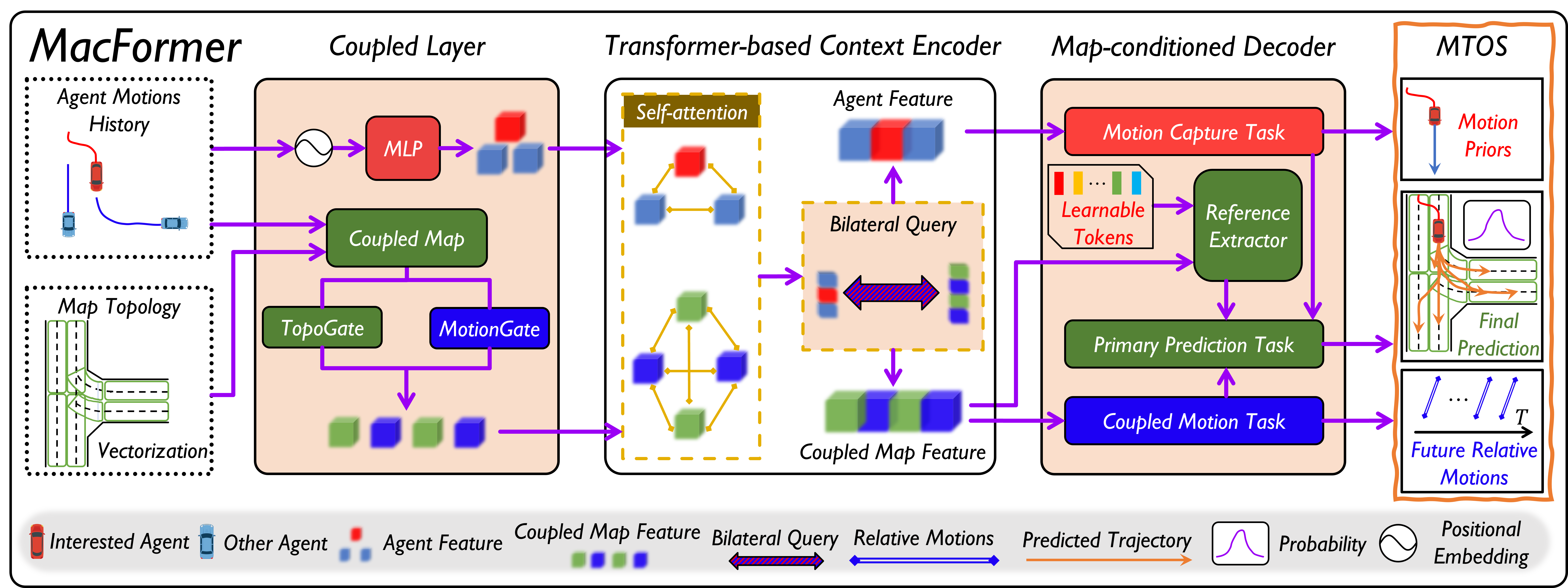 |
MacFormer: Map-Agent Coupled Transformer for Real-time and Robust Trajectory Prediction
Chen Feng, Hangning Zhou, Huadong Lin, Zhigang Zhang, Ziyao Xu, Chi Zhang, Boyu Zhou#, and Shaojie Shen.
IEEE Robotics and Automation Letters (RA-L) with ICRA, 2023.
We propose MacFormer, an one-stage Map-Agent Coupled Transformer for real-time and robust trajectory prediction
that explicitly incorporates map constraints into the network achieving state-of-the-art performance with significantly lower inference latency and fewer parameters.
|
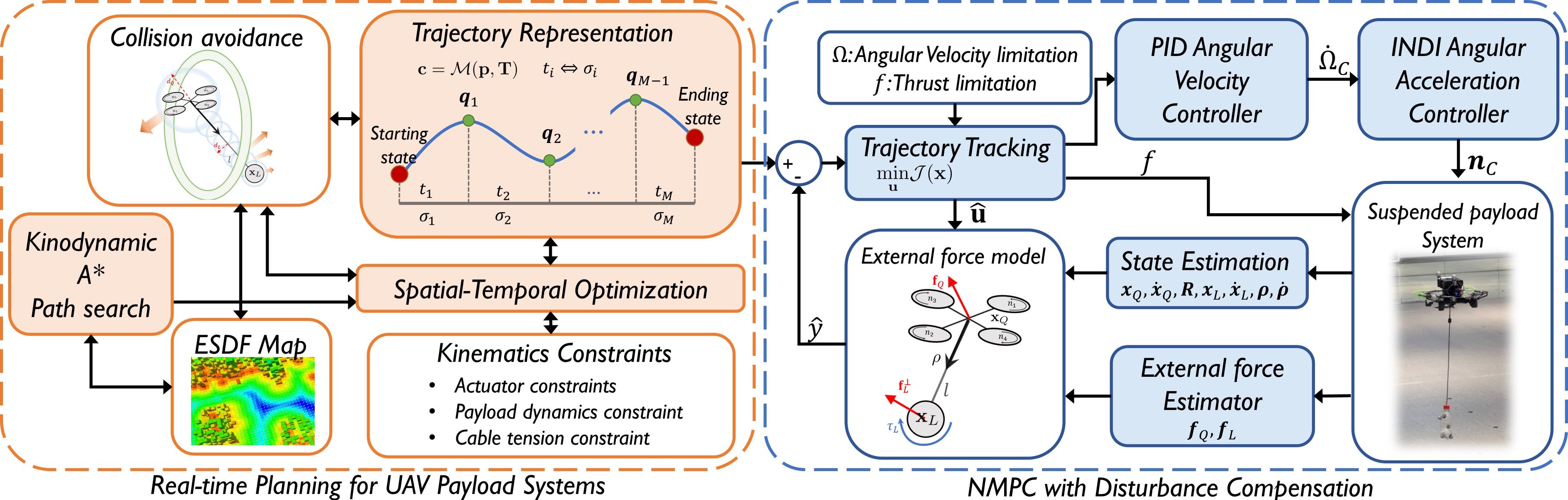 |
AutoTrans: A Complete Planning and Control Framework for Autonomous UAV Paylaod Transportation
Haojia Li, Haokun Wang, Chen Feng, Fei Gao#, Boyu Zhou#, and Shaojie Shen.
IEEE Robotics and Automation Letters (RA-L) with ICRA, 2023.
Star
We propose AutoTrans, a systematic solution for fully autonomous aerial payload transportation
that includes a real-time planning solution to generate smooth trajectories and an adaptive NMPC with a hierarchical disturbance compensation strategy to overcome unknown external
perturbations as well as inaccurate model parameters.
|
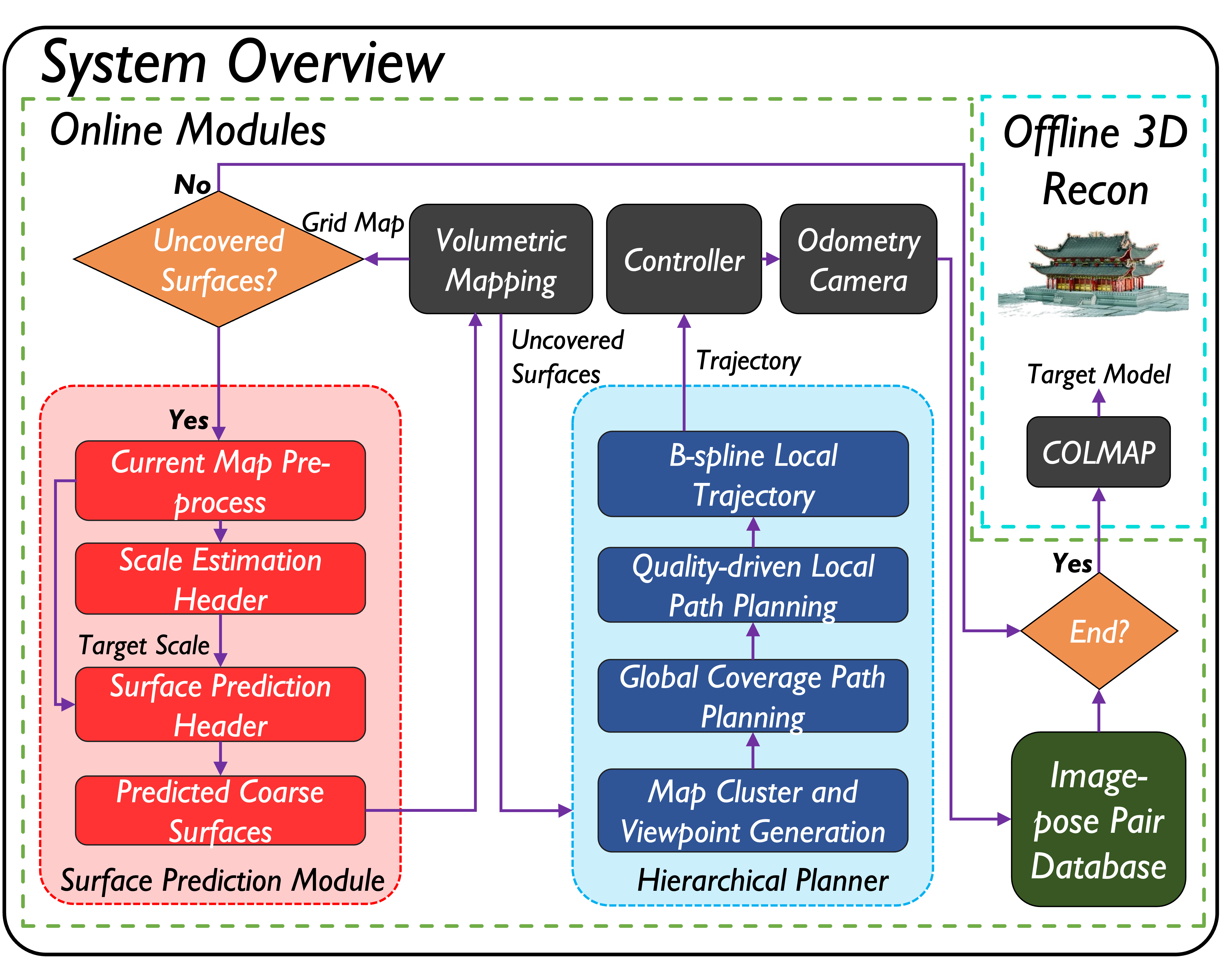 |
PredRecon: A Prediction-boosted Planning Framework for Fast and High-quality Autonomous Aerial Reconstruction
Chen Feng, Haojia Li, Fei Gao, Boyu Zhou#, and Shaojie Shen.
IEEE International Conference on Robotics and Automation (ICRA), 2023.
Star
We propose PredRecon, a prediction-boosted planning framework that can efficiently
reconstruct high-quality 3D models for the target areas in unknown environments with a single flight.
|
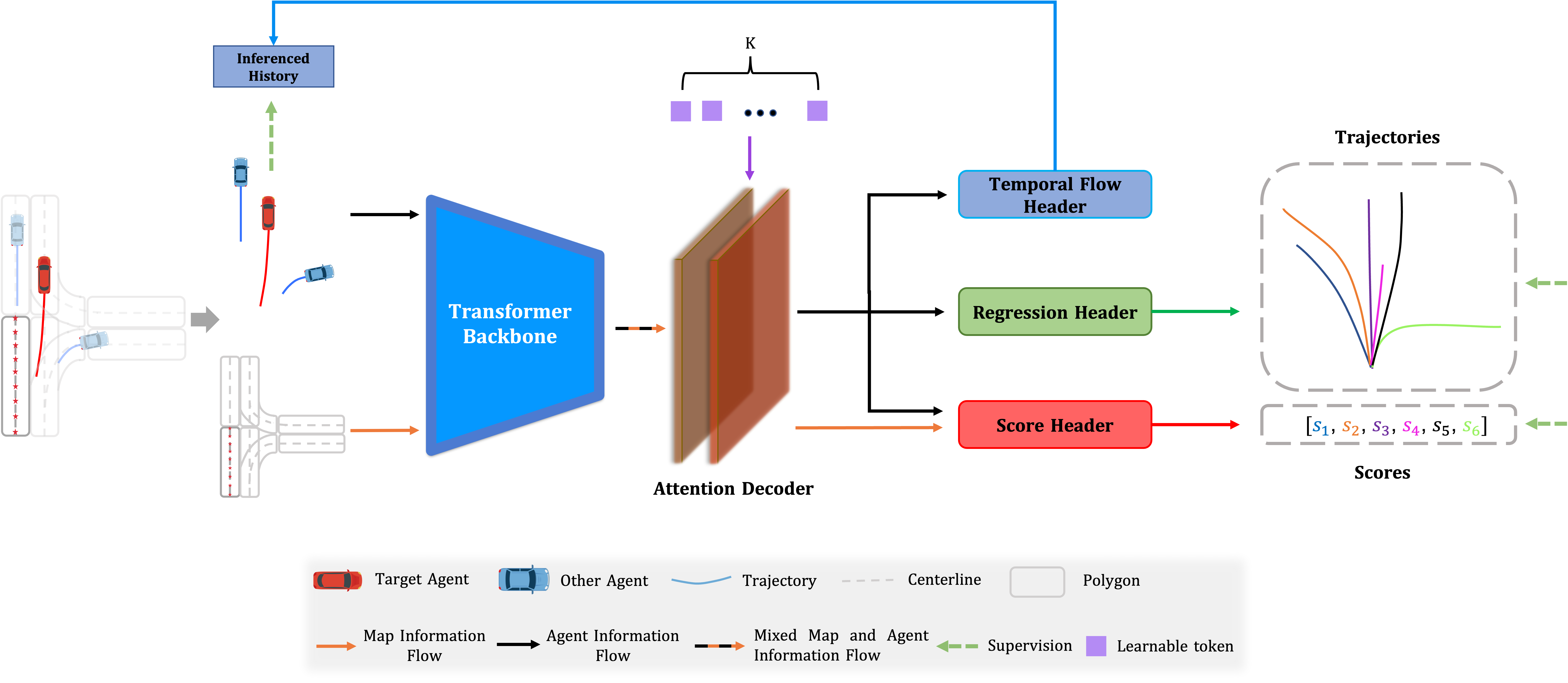 |
TENET: Transformer Encoding Network for Effective Temporal Flow on Motion Prediction
Yuting Wang*, Hangning Zhou*,#, Zhigang Zhang*, Chen Feng, Huadong Lin, Chaofei Gao, Yizhi Tang, Zhenting Zhao, Shiyu Zhang, Jie Guo, Xuefeng Wang, Ziyao Xu, and Chi Zhang.
IEEE/CVF Computer Vision and Pattern Recognition Conference Workshop on Autonomous Driving (CVPRW), 2022.
We propose TENET to enhance the trajectory temporal encoding via Temporal Flow Header. Besides, an efficient K-means ensemble method is used. Using our Transformer network and ensemble method, we win the first place of Argoverse 2 Motion Forecasting Challenge with the
state-of-the-art brier-minFDE score of 1.90.
|